Juniper Networks Enhancements to Marvis VNA: User Data, ChatGPT, and Marvis Actions

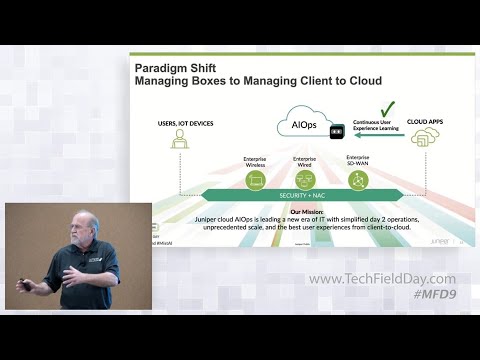
Juniper Networks Enhancements to Marvis VNA: User Data, ChatGPT, and Marvis Actions
Marvis, the industry’s first AI-Native Virtual Network Assistant (VNA), has grown a lot over the past year. Join Bob Friday, Juniper’s Chief AI Officer, to get an overview of Juniper’s latest Marvis innovations and what they mean for your network operations.
You’ll learn
The key components of Marvis
How Marvis is advancing, including continuous user experience learning, LLM, Marvis Actions
Who is this for?
Host


Experience More
Transcript
0:09 I'm Bob Friday TPA officer Juniper CTO of junipers Enterprise business it is
0:15 great to be here I think I know most of you you know for missed in The Marvelous data science teams this is the event of
0:21 the year you know this is what we work towards every year this is we come to be at and for me personally I'm known for
0:27 saying Dentures like Miss are the ultimate team sport Wireless networking is a very small world I think we all
0:34 know each other from everything and I want to personally thank everyone here because the Venture would not have been the same without you you guys are part
0:40 of the team we got here with all your influence and all your knowledge of the industry so thank you very much
0:46 it is a team sport you know this adventure started when Susan and I were at Cisco and I think
0:51 you guys have heard this story before basically we were there when we were hearing from some of our very large
0:57 customers where basically they wanted us to have the controllers to stop crashing they retire that they also wanted to get
1:04 things quicker than a year right they want us basically innovate faster and keep up their digital transformation and
1:09 probably thoroughly and most importantly before they were going to put anything critical on the consumer device like an
1:15 app or on the network they wanted to make sure that it was going to be a good experience
1:20 and this is what I would say is the foundation of Miss was really this Paradigm Shift we still have to keep all
1:25 these boxes green you know AP switches rare all those things have to be running but the paradigm shift is really around
1:31 AI Ops moving us from my client to Cloud tradition right we want to make sure that that connection is going to be good
1:38 and what you're hearing here is one of the big new announcements we're going to be making today is really bringing this
1:44 Zoom data into Marvis and this is really around how we're going to start ingesting data
1:51 labeled data about your user experience and why this is so fundamental is this
1:56 is basically going to let us to start to leverage Technologies like chat TPT and taking terabytes of data training models
2:03 that can actually predict user performance and start to understand why you're having a poor experience so this
2:10 is a big announcement this is something I'm very excited about and I think the team in this data science team is very excited about
2:18 you know this journey I think you've heard this story before when it comes to AI it all starts about the data you know
2:25 I'm famous for saying you know great wine starts with great grapes great AI starts with great data right and that's
2:32 why we've always started the journey with data why we built access points to make sure we can actually get the data we want what we're doing today today
2:38 around Zoom is really starting to extend that data thing right we're always been ingesting more data more data lets us
2:46 actually solve more problems you know what you saw when we started the journey we started the journey with wireless
2:52 access points because that's what allowed us to answer the question of why are you having a poor internet
2:57 experience right and what you saw us do at Juniper was basically extend that across the access point the switch the
3:05 router and when we got to the router that's what let us start to answer questions about the application layer
3:10 you know and what we're doing with the zoom data is now is to start to answer questions why are you having a bad Zoom experience and when you talk to our
3:17 customers you'll find that usually that is in the top three support ticket problems right you know when you think
3:22 about Zoom teams collaborations that's like the canary and the coal knife
3:28 right that video application is probably the hardest application to run on your network if anything's going to break first it's going to be your video
3:34 real-time video collaboration experience so that's what we're focusing on here is really starting to understand that
3:40 Canary and getting data from it probably the second big thing in the data science you know we all have access to the same
3:48 algorithm right what makes a difference here is a data science team
3:53 you know and I think that is a time you see with Miss if we've been working on this ever since this started and what
4:00 we're announcing this year is really in addition to the data science team we've added new data members to the data science team and one of that is really
4:07 around observability and we're going to be talking a little bit more about shapley and I know if you've heard of shapley
4:13 this is really a technique to understand why a model is making a prediction So
4:18 This falls into observability and helping you understand that Zoom experience why are you having a bad
4:24 zooming performance if you can predict someone's Zoom performance you can now understand why that performance is
4:30 either good or bad and that is shapley that's what shapley is doing for you and then finally on the conversational
4:35 interface for me I personally believe the conversational interface is the new user interface for
4:42 not just beyond networking right I think we're seeing with chat gbt how we get data out of systems is changing we all
4:49 started our experience with CLI 20 years ago turkey the dashboards
4:54 Next Generation University is going to be these chat gbt conversational
4:59 interfaces and you're going to see us being integrating that in our conversational interface making it easier for you to get information and
5:06 get to the data you want quicker and then finally on actions this is sadir's favorite part you know Bob forget about
5:13 all this fancy math it's all about what can you do with it right and this is the sound driving piece and you'll have
5:18 three new announcements on how we're helping the self-driving part of Marvis going forward
5:26 so up to today you know what you've typically seen is in Marvis two key components right there's been the
5:33 conversational interface around troubleshooting helping you get to what you're seeing in the network and that
5:40 starts with what we call AI events and insights right you always have had access to the raw data you know that's
5:45 where it starts taking these raw events using AI to get them filtered into something more actionable
5:51 conversational interface marvelous actions has been the self-driving piece of Marvis this way it actually lets you
5:57 basically start to offload your functions right this has been the bad cable story self-driving RMA tickets you
6:04 know where you start offloading responsibilities to Marvis the new piece that we're announcing today is around
6:10 this user experience continuous learning this is where now Marvis will be taking
6:16 data continuously real time from your public Cloud apps Zoom teams video
6:23 collaborations continually see watching that user experience and using that label data to basically build models and
6:31 predict because once I can predict your performance accurately I can now understand why you're having a bad
6:37 experience so that is the power of AI ML and using terabytes of data to actually
6:43 build models that actually can actually predict events question
6:48 so one of the things I've always thought is that defining good Wi-Fi from metrics
6:54 can be a little bit of a challenge and having user input might be helpful in the sense that a
7:01 user can report that they're having a bad experience in their perception which can often be
7:06 fought is that something that you guys are considering some sort of like pay right now my Wi-Fi is bad kind of button
7:13 that someone could use to re report and then you would get capture the data and then maybe use that to help train the
7:20 model going forward yeah I mean so what we're talking about here John is reinforcement learning yeah so when you
7:26 look when we when we'll talk about this more in detail exactly how this Zoom model works but the answer is yes this
7:32 is reinforcement learning taking human actual feedback into the model and basically making that prediction better
7:39 right so the answer is yes and this is the power of ml right in that you're seeing it with chat you mean if you look
7:44 at the chat GPT you'll find that human reinforcement learning and that is what made the difference between chat GPT and
7:51 the other models given the almost ubiquitous presence of teams and zoom clients on all laptops
7:58 and devices out there we're leveraging that uh you know user sentiment directly
8:05 into the Miss dashboard I'll show you a demo yeah so so in sort of pushing yet another agent that they have to download
8:10 and say Wi-Fi is good or bad every Zoom call every team's call usually we don't
8:16 we don't click that happy five star button if you're happy but we know when we don't like it we definitely click it
8:21 right when we have a bad call so we're leveraging the install base of of clients out there to actually provide
8:27 this what's pushing a net new client and if I live long and strong you're going to have a little harvesting on your Zoom
8:34 as your Zoom call someday okay uh so that is the basically a new
8:39 fundamental component to Marvis you know this user experience feedbacks component this will be something that will be
8:45 running continuously in Marvis going forward
8:51 now this is my AI slide you know what I try to tell people is AI is a concept it's about
8:58 the next evolution in automation we've always been doing automation with scripts AI is just taking that
9:04 automation to the next level doing things on par with humans right and when you look what's going on
9:09 when you try to do that it's not just one algorithm like that self-driving car these are all the algorithms that are
9:15 under the hood of Marvis right and these are the benefit when you'll actually go down and actually look what's going on
9:21 it's the upper right hand corner that's disrupting right the algorithms down here the
9:26 machine learning this is stuff we've been doing for 20 30 years all part of the picture but what's going up in the
9:31 upper right is what's transforming the industry right now Transformers right you see what's happening those were
9:37 introduced in 2017. that's what's the underlying technology underlying chat
9:42 GPT you know the lstm models those are the models that basically let us get anomaly
9:48 detection to be reliable right you know that was our journey around how to get an army detection and generate fewer
9:54 false positives so this is all a key part you know for people in the networking World on AI the
10:01 mission here is ai's evolution of automation uh there's a lot of moving
10:06 pieces going on underneath the hood the other key part I think I've reached
10:13 before is I don't think people fully appreciate is yes you know as sujay reminds me you know when we left Cisco I
10:20 didn't say we didn't have ai in mind what we had was real-time operations you know how do we help with real-time
10:25 operations you know AI came in later but the other key part that when we left was
10:31 really organizationally you have to get the data science team connected into your support team
10:36 and when you think what's happening with Cloud AI once we take all the data to the cloud
10:43 our support team is a proxy for our customers right now the fewer tickets our support team
10:50 sees is basically the fewer tickets that our customers see and so when you see it Miss and I would
10:56 say this is probably one of the biggest differentiators that Miss and I would challenge anyone to talk to our
11:01 competitors if they're not using their own aifs tool in their own operations team they're not there yet this is part
11:09 of the journey and we've been on this journey since 2018. what functionally what does that lower
11:16 bubble mean right does your does do your customers talk to the data science team
11:21 directly like like what does that actually mean so what this actually means is there's a person like a person
11:28 named David or support team our customers talk directly to David J right there's a connection right there when
11:33 our customers have a problem they call them David J now David J meets with that data science Team every week
11:40 right so every week they go through all the tickets and they figure out which tickets can actually AI help with
11:47 now those customers we meet with these customers probably once or twice a year and they talk to us directly on what
11:53 they tell us to do what what they want every year so what that means directly those customers doctors twice a year
11:58 those customers talk to David J Daily weekly David J talks about data science
12:04 team weekly to go through all the support I'll be honest um when Bob first put this Pro you know
12:10 concept out there it didn't feel big it didn't feel okay what's the big deal about this right so uh but if you go to
12:17 the next slide today after five years of every week looking at every ticket that
12:24 comes in and saying did Marvis have an answer did Marvis have the data did I
12:29 have to go into the cloud did I have to go into the back ends of the cloud or do I actually have to go to the AP to get
12:34 data those are the five categories here by by virtue of putting every ticket
12:40 through that standard we were able to continuously try and focus on making
12:45 Marvis have the answer right just to say organizational focus on every ticket
12:51 that comes in so technically as a proxy our customers are talking to us with every we support ticket they open and I
12:57 would tell you up here this this top color up here this yellow cover that represent how often the support
13:04 team had to go SSH into the AP or the switch to get data
13:09 and so when you're on this journey the first thing you have to stop is all sshing right need to get the data to the
13:16 cloud whatever problem you're trying to solve the data wants to be in the cloud if you're going to solve it with AI and I would tell you most of our
13:23 customers saw value forget the aips they sell value in the cloud just getting the data to the cloud was it was a
13:29 relevation because now once it was data in the cloud they could solve the problem itself once they had the data where they needed it so that's the first
13:35 part of the journey you see it took it a while to get that done right it's culturally hard to stop people people
13:41 who've been sshing into boxes for 20 years it's hard to tell them to stop that right that they had to stop that then
13:47 plus we had to get the data with that by itself was hard to get the data out hey Bob I have a couple of questions
13:53 um with with Marvis now is the interface to Marv is going to be a chat gbt
13:59 experience is that what is it kind of replacing the user experience and then my second question follow-on question is
14:06 that GPT has a learning ability that they can learn from the person that's entering
14:11 are you now going to start capturing knowledge and information from your customers and what are the implications
14:18 of that I think the implications of the question around the answer is yes we're going to be integrating large language
14:23 models Into The Marvelous conversational interface it's going to start with the knowledge base as opposed to you know
14:30 we'll move on to networking the first mission will be knowledge base what the the large language models will do they
14:35 will not for say captured data but they will be much more of a dialogue experience those large language models have a very
14:42 large extended input they take thousands of tokens so as you communicate with it it remembers what your what you said
14:49 before that's a little bit different than reinforcement learning so that conversation will be a little bit more
14:54 human-like a little bit easier to get data out of you're getting data out of documentation or start to explore your
15:00 network data oh okay so if I have a certain way of
15:05 dialoguing when I log on it will then start using those terms when it's
15:11 explaining things to me is that what you're saying it's like learning my language it will start to learn your it
15:17 will start it will with reinforcement learning it will start to learn your language okay you know as we start to
15:22 actually incorporate reinforcement learning and training the models basically being more domain specific
15:28 okay okay and will it sorry I had to drag on this but will it you know if I
15:34 start asking questions my network is doing this why is it doing this is Marv
15:40 is then learning that my network is doing this and and then making that part of the
15:46 knowledge pool that then can be shared with other customers that is where we're headed and so that is the power of these
15:53 models right right right and basically to become much more personalized to your network yeah right now where we're
15:59 starting right now this will become a knowledgeable over start will be knowledge basis it'll be a language model that's very Juniper domain
16:06 specific so you can have to start answering questions about General domain specific stuff right okay but where your
16:12 head is eventually yes we'll head to the point where this model will get very specific about your network right great
16:17 okay thank you good thing on that like So eventually sharing with the customer right so customer X for example has
16:25 let's say AP 45s and certain clients certain code versions that they're running and let's say something happens
16:32 and the clients are experiencing some some issues of problems or something like that because of certain whatever reason customer y has a similar setup so
16:41 will that information somehow be displayed or did that other customer
16:46 also to possibly they can figure out uh not now these are separate I mean right
16:52 now right now we'll talk about this there's a whole section on the on the model we get to I would say right now
16:58 what's happening these llms we're fine-tuning them for Juniper domain so
17:03 this will be a juniper model specific to Juniper knowledge uh that same concept
17:09 can be extended down to customer site and org right you know we could start organizing llms that are
17:16 customer specific which is not interlapping that'd be like a customer
17:21 to have a specific model optimized for their environment right for your network
17:28 well if I may ask a question about your chart is this you talk about SSH and people having to SSH into the devices is
17:35 this to identify a problem with the AP or is this looking at just like hey the wireless clients are having issues yes
17:41 and yes yes I mean people that says H is in the boxes all the time so there's gave me move on look yeah no no no no no
17:47 I I just also want to add that um yeah so basically these are support tickets
17:52 as they come into our uh on our our uh our support team right just anything hey
17:58 you know an AP can't reach the cloud my my users are having radius issues and I looked at radius everything looks okay
18:04 can you help me just anything right people are complaining about Zoom calls every ticket we put through this measure
18:10 and so what the green is saying is you know when we Mark a ticket green that
18:15 means Marvis actually had the right answer um blue says Marvis had the data the answer wasn't you know exactly what we a
18:22 human envisioned it to be and and so the the grading goes like that and the the interesting thing is if Mario already
18:28 had the answer why couldn't the customer self-serve themselves right and so they do so each week the problems become
18:36 harder each week if I saw the customers can sell sure they're not going to open a ticket the next week it's the
18:41 different set of problems and so this will be a journey that will continue to be going up and down like this because harder and problems are harder every
18:48 every week I mean you see things you see we still SSH it there's still problems we find that they have to go into the AP
18:55 or switch or something to find out what's going on you want to ideally you want that to be zero you want this green
19:00 to be way up here you know that's the journey we're on now it gets harder and harder to get down to get the little
19:05 hanging fruit out of the way now we're down to the harder harder problems that come up
19:12 okay now we're going to get more into the continuous learning experience I'm going to go a little bit deeper
19:18 before I hand it off syrup for the demo you know if you look what's happening here you know what we're doing here is
19:24 we're taking real-time data from Zoom this is session data per minute session
19:30 data we're getting from zoom we're joining that data with network features now this by itself has value so there's
19:37 going to give you a demo if you now want to go look to see what's going on with your Zoom performance relative Network
19:43 features uh it teams will now have access to both Zoom data and network data on the same
19:50 graph that starts to help it teams start to troubleshoot problems we're going to get these Zoom calls in what we're doing
19:56 here more specifically though this is where we're leveraging catch EBT we're
20:01 taking these deep learning models they're taking terabytes of data we are now training a model that can
20:09 predict your Zoom audio video latency your Zoom performance
20:16 now once you get that accuracy up there and we're getting down to like I can now predict your Zoom latency plus or minus
20:22 five milliseconds ninety percent of time think about like going to a circus and trying to get someone's weight you know
20:29 how accurate can we do that the more accurately I can do that I can now use these techniques called
20:35 chat blade I don't think it means Twitter shapley but shapley is basically a data science technique to start to explain the
20:44 features of a model so when you get down to this graph here's what they call shapley graph
20:49 I can now explain which network feature is responsible
20:54 for your latency right so this starts to let us get down to figuring out whether or not is it a
21:00 wireless problem or a Wan problem or a client problem and the good news is when we get data
21:06 from Zoom they start to give us information about the client turns out maybe 10 20 of the problems are have
21:12 nothing to do with the network it has something to do you're downloading a video while you're trying to do your
21:17 Zoom call you know and they have visibility in that they know if that laptop is overloaded the CPU is being overloaded
21:23 while the zoom calls are going on so this gives you more visibility of what's going on through this client to Cloud experience right are you able to see
21:30 like hey your antivirus is running and it'll say this processes it didn't tell us what process it'll tell us what CPU
21:37 it'll tell us that basically the CPU is overloaded right now yeah you forget other The Marvelous SDK is
21:43 starting to get more visibility in what's going on on the on the laptop but the zoom information gives us basically
21:48 CPU information what's going on a laptop okay I'm gonna turn it over to another
21:54 question I'll give it over to Senator is going to give us a quick demo let's get back to the real world so
22:01 um uh before I go to the demo just very quickly the net net summary of this slide is if your organization has uh is
22:09 running Zoom right now we're launching with zoom teams will follow it later this year um but if your organization runs Zoom
22:16 right now we could actually connect the zoom instance to the missed cloud and
22:21 and be able to start to get you know uh where and what problems what network features are causing problems but the
22:29 the true power of this entire thing is even if you don't have zoom in the
22:34 future our ability to predict based on the network features we see our ability to predict what uh if your network is
22:42 capable of running a audio video stream right now so let's look at it so uh basically
22:48 um you know what what uh what you're seeing here is We Are For the First Time natively integrating uh Zoom call data
22:56 in here right right so directly from Zoom Cloud on a minute by minute basis you know latency packet loss Jitter
23:03 coming in and audio in audio out video in video out screen share in and out all
23:10 of this data is is coming from the zoom Cloud into the Mist cloud and average van latency here is the Network's
23:17 assertion right now stacking the networks assertion of what the latency looks like and to zoom's assertion of
23:24 what latency they saw from the client to the cloud right connecting these dots and be able to actually then take you to
23:31 prediction this is we're going to put our homework there our prediction of what we thought the zoom call should
23:38 have done versus what it has done right and this is what Bob's talked about in shapley and what uh what chapli has done
23:45 here is is be able to basically you know show you um you know
23:51 um this this whole whole you know on on a feature by feature basis right so
23:57 which features we believe so just to give you an order of magnitude example right one of the customers that we've
24:03 connected their Zoom instance to our missed Cloud they're doing 20 000 Zoom meetings per day right now
24:10 right 20 000 Zoom meetings because again if you think about it they're about a 25 000 employee company 20 000 Zoom
24:16 meetings is nothing it's like one meeting but for us we now have 20 000 Zoom calls times the number of minutes
24:23 that they've had the call and put all of that data and say across all these 20
24:29 000 calls in this organization what were the what's commonality what's the probability of what caused maybe a
24:36 certain radio channel maybe all the people on a certain Channel were the ones that experienced failure maybe
24:41 someone uh you know with with a overloaded APS this this whole you know
24:47 uh sharply model is going to take us to another level now take this forward
24:52 Marvis now you could say hey at this organization level show me the zoom
24:58 calls that happen right being able to connect the zoom calls natively into the marvelous conversational assistant and
25:04 say okay you know at this site I mean how many calls have happened you know and and who's actually being been on a
25:11 zoom call and this Green Dot says that was a happy call The Green Dot says they the user had a good zoom experience and
25:19 so consequently now not only are we able to connect the zoom experience we're connecting it end to end client to cloud
25:25 from uh the client to the AP to the switch to the van edge up into the zoom
25:31 Cloud that entire experience we are able to uh collate it but the power of this
25:37 thing is just ask hey were there any bad Zoom calls how often have you had a CEO
25:42 have a bad Zoom experience you know that kind of stuff so here we're saying ah Kumar had a bad Zoom experience and and
25:49 you know why okay okay that device is roamed in the middle of the zoom call right powerful connection between the
25:56 network and the application on a minute by minute basis only a cloud enabled Cloud native microservices architecture
26:03 can do this right now let's go look at hey what was Kumar's laptop's experience
26:08 look like on the wired net wireless network site right you know troubleshoot that particular MacBook Pro Boom the
26:15 network said hey this device is doing an interband ROM and consequently you know
26:21 uh in the middle of Zoom call so think of all of the Hoops you have to run
26:26 today to be able to actually connect these two simple things and and uh John
26:32 to your experience yes we are bringing uh the zoom uh you know rating natively
26:39 into the cloud here so um so basically you know not only is the user feedback
26:45 captured and that becomes you know when you say show me bad Zoom calls you know that becomes the green or red dot uh but
26:51 this is the audio quality from Zoom as as determined by Zoom not the user video
26:57 quality by Zoom as determined by zoom and and Screen shared quality this is the human feedback all of this
27:04 connecting the dots with uh the actual end user experience right so um we're
27:10 super proud for two reasons uh I think this is uh this we are super excited
27:15 number one it just makes Wi-Fi troubleshooting uh for these types of applications easier that's that's the
27:21 that's the low hanging fruit that's the low bar the reason I'm most excited this is for the first time we're getting real
27:27 labeled data for machine learning models to actually have had a granularity that
27:33 matters on an interview user every user every device every Zoom call every minute basis this is labeled data if you
27:40 think of what chat GPT and that team has done open AI its labeled data is is the
27:45 power of that thing so Bob with that uh let's uh let's talk about uh llms and I
27:51 would say you know for those who want to actually go down the rabbit hole in this the uh the missed data science team is here today so during the break feel free
27:58 to you want to learn more about shaftly it is a very cool thing to actually have how it actually works uh this is the
28:04 other cool piece we're looking at is you guys all saw chat GPT this is basically I think if I have any
28:09 AI Skeptics left I think it's starting to get my skeptic crowd guys this guys is going to make a difference uh we've
28:15 always been a big fan of conversational interface uh we are going to integrate LM into our conversational interface but
28:23 the blue shows you is what we've had up to date right it's been mostly around nlu right we've been able to take your
28:31 questions understand the intent of your question and try to answer it what we're
28:37 adding in the green so that's what we had today that's what we've had for since 2017. what we're announcing today
28:43 is what's in the green and when you look at these llms there's two approaches to them
28:49 one is basically what they call Prompt engineering where we do a somatic search take that
28:55 and basically try to get an answer out of this and it's what prevents hallucination right so what we're doing
29:01 here we're not going directly to chat CPT we're basically taking Juniper documentation
29:07 indexing it and then prompting the llm to give us an
29:12 answer for you so what you're going to be seeing going forward from today is basically in
29:18 addition to just getting docks you will now get a natural language answer so this is basically nlg
29:24 so we've had nlu for years what we're really starting at is nlg
29:30 now what you look forward in the green going forward dialogue data exploration
29:35 as we start to fine-tune these models either the open AI model or the open
29:42 source models this is where we get into we can start fine-tuning these models even down
29:48 to more specific problems and so we'll start fine-tuning models for more Juniper questions that can eventually be
29:55 taken down until we can even fine-tune models for customers right if you want to get the model to be very specific to a specific Network and you fine-tune
30:01 these models and train them on a specific Network customers Network so
30:07 this is what I'm excited about this you know question and as the documentation is the input
30:13 for the learning model are you going to review and update the documentation as
30:19 well Sadir has promised 1 000 documents by
30:24 so the question uh uh Raymond uh is is are we improving our documentation to
30:31 augment all of this AI absolutely in the last one month uh literally we've we've
30:38 done you know Mist has been great at many things in the world documentation is not one of them right and so uh we've
30:46 taken that seriously in the last month the month of April uh significant progress on all of it all of the Realms
30:52 wired wire Wireless van Edge all that kind of stuff yes and and it was a wake-up call every video on Mist we now
31:00 transcribed so that the llm models can consume the transcript uh and they don't have to consume a video per se so yes uh
31:07 absolutely um the major investment for us so you're seeing the light llm has brought a big
31:13 light to documentation because yes you know for IAI you need data Ln
31:19 the documentation is funnily the data that data's got to be good garbage and garbage out so we gotta get that data cleaned up and actually make sure that
31:26 the the contents there that answers your question and then a question on the the query and then the output is that
31:33 contextual to would that be contextual to the user as well would the Peters of the world get more deeper technical
31:39 information versus like one help desk Tech let me think about that the is it
31:45 contextual to the level yeah level so yeah so if you've got if you've got heater making having a question versus a
31:53 help desk Tech having a question does Peter get more information than the help desk uh the answer is no not now though
32:00 you can train it that's what the fine-tuning we could train fine tune for either a you know a new person versus an
32:06 experienced person that is possible it's not being trained to do that yet uh it is trained right now right now it
32:14 is contextual in the sense that it's contextual to the equipment that the customer owns you know if a customer has
32:21 switches and routers we won't answer data center questions unless they have data center equipment
32:27 and so we try to make sure that it's contextual into what the customer actually has running in the network
32:32 so building on that does that would it because it is learning theoretically
32:37 um like chat2pt does it learns over time will it will it'll kind of predict what I want
32:45 or what's being asked of it in certain circumstances so it kind of diving deeper getting more information that
32:51 might be relevant to the askers sort of building on that last question which I'm guessing it doesn't do yet but if I
32:56 always ask it X followed by y yeah I would say I mean
33:02 the element model itself is basically just a pure probability prediction machine it's not personalized to you per
33:08 se it is personalized how you answer create prompts and so you can learn how to get it to
33:15 answer questions in different ways though I would say that you know you look at it's really a big probability
33:20 model um it's not it doesn't know who you are but it is sensitive to the prompts in
33:26 that sense it'll feel like it's personalized right to pay how you ask prompt and ask questions I think the three things I'd like to
33:32 kind of take away from is you know what you have today nlu conversational
33:37 interface that's what's there today what we're announcing today is summarization of public documentation what you're
33:43 going to start to see from us is a much easier way to get knowledge out of the Juniper knowledge base
33:49 with Cedar's new documentation initiative what you're going to see is head to is basically more personalized
33:56 fine-tuned llm models and down to we can fine-tune them either to Juniper or fine
34:02 and tune down to the company org so so a quick question um I'm seeing a potential for this to
34:08 help with the documentation issue as well because with with the missed product it's updating all the time and therefore
34:15 I think that's the challenge of keeping documentation if I ask this a question and it tells me to do something go somewhere and that
34:22 doesn't work and I say that didn't work and will that therefore be able to
34:27 provide an input back into the documentation instead of documentation was wrong and therefore update yeah this
34:34 that reinforcement learning algorithm the answer is yes now we're starting with our support team
34:40 because our support team representative but that can be that will also be customers customers give us feedback that will go back in that reinforcement
34:46 algorithm and and the short answer actually if you go to the next slide Bob uh uh Peter is uh this dialogue gives
34:55 you that opportunity to so so what we're launching today is a one-way summarization but uh where we are headed
35:02 is the dialogue that says I don't like that answer just like the chat apt you get five answers you could choose which
35:08 one um you that is that is where we want to go right we want to learn from our Rich
35:13 user data set yeah yeah because I think that's important because it's something I found is that you know an answer may
35:20 have been true a month ago but it's not necessarily true today it's right that's right yeah and also to Chris's point I
35:25 think personalization could be could be very very interesting right so
35:31 um thank you for that and here's a very very quick view of um what uh the the uh
35:38 chat GPT integration into uh The Marvelous conversational system could look like uh essentially uh the idea is
35:45 uh you you ask you know a documentation question we are now polling that you
35:51 know prompt creating using Juniper context and asking out the question off of uh the chat GPT and bringing the
35:59 answer right in the previously like Google we would throw up a bunch of links but now we have summarization uh
36:06 this is this is what is new there's immense potential this is the very first
36:12 step in a very rich long journey that we are ahead of what we are proud of is in
36:18 2017 when Bob told me we're going to launch conversational assistance and you know everybody competitors tweeted
36:24 saying oh wow look at these guys can't build dashboards now building conversational assistants you know we
36:30 were five years ahead of chat GPT that rich data that the framework and the micro Services Cloud architecture we
36:37 have built we feel uh is just hand in love to what llms have brought for us right so we're super stoked about uh the
36:44 possibilities here this is the early rendering of this how far are you going to take the the the end user education
36:51 side of that conversation because if somebody continues to ask at some point they're going to run out of your documentation right if somebody says Hey
36:58 explain to me what the OSI model means that's right that's probably not in Juniper's documentation because you
37:03 expect everybody to know that at what point are you going to stop supporting those users and say sorry you got to go
37:09 somewhere else for that answer or are you going to lead them with external resources to Wikipedia or some garbage
37:16 like that so yeah so if it's not you know be careful if it's not in our knowledge base we will go to chat
37:22 because if you look at Chachi PT itself or GPT 3.5 it basically can answer
37:28 Junior network engineering questions I mean if you look closely
37:33 I have a question um so when I use chat TTP to summarize
37:39 anything it kind of mixes up the data how do you secure that it doesn't accept the documentation for your products uh I
37:46 mean so what we're doing with the prompt engineering you know when we're doing somatic search on the doc searches the
37:51 answer is limited to our documentation now there's still issues of it can still
37:57 kind of mixed up even so it's not going to hallucinate it's not going to pull stuff off the internet when we do that
38:02 no but still if I have like a threat of three videos transcribed uh it will mix
38:09 them up uh so it will change like company names or titles and stuff like that yeah
38:15 they're they're still when we when we feed in the prompt into the LL end yeah you know right now we're looking at
38:21 feeding in one document at a time so but it can still pull parts from the top of the document to the bottom of the
38:26 document the reinforcement learning is what's going to fix that okay so if you look at the train in the model there's
38:32 the first piece of it is self-supervised reinforcement learning where we're doing a support team will start to eliminate
38:38 that type of problem and that's the full loop of training these models to get better answers yeah in my view two
38:44 simple ways in which we will avoid hallucinations number one is the art is in the prompt creation and and the
38:51 associate reinforcement learning that's number one number two there is absolutely human data labeling that we
38:57 are pursuing chat CPT also pursued and so first The Prompt creation with
39:02 juniper context and second you know we're labeling as as fast as so that we
39:07 can give that reinforcement to the model yeah but all ultimately there's a probabilistic model ultimately the net
39:13 we're not going to be replacing Network engineers and ultimately someone's got to look at the answer we're trying to help them get to the date the answer
39:19 they're trying to get to quicker faster I think the other big thing you know in addition to zoom and LTE we're
39:25 announcing three new marvelous actions and we'll talk about two of them here right now one of them is wired broadcast
39:31 anomalies this really started with a customer higher ed customer he was deploying the network
39:38 um turn out he was deploying a very flat Network I think he got up to three or four thousand APS and all of a sudden
39:44 the network crashed right you know so what we've done now is basically put in a model that looks for
39:51 broadcast storms multicast packet broadcast storms so this is an
39:57 extension of our lstm anomaly thing and this is where lstm models have
40:02 actually made an army detection useful and practical where we have very low false positives if you look what's going
40:09 on here at this piece of the puzzle what this model is predicting is really distribution functions
40:15 you know when you think about ml models you think about them trying to predict a single point like your your age or your
40:22 weight or something right the fancier these models get they're not predicting just
40:27 how many multicast packets you're going to have on the network they're predicting the distribution
40:34 right and this is what's allowing us now to basically detect these broadcast storms before they bring your network
40:41 down so in that use case there were you know they kept adding APS you know if
40:46 they had had this they would have known that you guys are having a problem right your broadcast traffic is getting up to a point heads up something's about to
40:53 break so we're very excited about this is yet another marvelous action this will basically prevent your network coming
40:59 down or give you heads up that you're about to have a broadcast you know you're reaching capacity problems
41:04 um the other quick thing we're looking at here this came from a customer where they had video cameras they're basically
41:11 had video cameras getting stuck on things either the switch was getting stuck or the camera was getting stuck
41:17 and they wanted a model that basically could detect anomalies on the actual Port whereas before we're attacking
41:23 network-wide broadcast storms here we're down to actually detecting a port is in trouble
41:28 and so this is detecting you know video cameras you know also in the video camera traffic has stopped now the
41:35 rumination that is either we reboot the port or reboot the camera so this is another little action that's coming down
41:41 for people who are actually running big networks with a lot of video cameras or devices that
41:46 may have to be rebooted on those devices and when you look at here the thing here is this is basically a tribute to the
41:53 team you know these are not I would say fancy deeply these are regression models with
41:59 a lot of domain expertise that have been built with a lot of work with the customers what about something like okay if it
42:06 detects an anomaly um AI can learn okay so certain action is happening is causing like a certain
42:13 problem well instead of me having to just constantly do it well let's just do it automatically right maybe if it's
42:19 like an approval button or something like that so it just happens automatically send a notification hey this is what happened and we just
42:26 rebooted your bounce report for this camera for example or that device and I think that's some more of action I think
42:32 we're getting to the point now you know if you trust Marvis enough we'll just reboot it for you you know he gives
42:37 permissible reboot it and then see we'll give it a quick demo yeah and and uh Ali I think uh in general that's where we're
42:44 headed it's capable today um but people also still have
42:50 consternation on Don't Touch My ports right and so so um it's very capable that's why what we
42:56 do is uh some of the customers that have done automation uh as an example uh you know we'll talk about uh what a launch
43:02 we did with service now uh in the partnership where they'll take these marvelous actions as web hooks they will
43:09 call an API to actually do the port but in their control so then they could get an approval email and saying would you
43:16 approve this action this is what Marvis detected so completely automated meaning Marvis rejected the action web hook went
43:21 to service now created the approval someone said just okay approved boom it takes the action that closed loop today
43:28 many of our customers do right and that's where you know the real-time uh uh sort of uh web hooks notification is
43:35 super helpful uh these are this uh traffic anomaly again we follow our
43:41 support tickets right as you saw every single support ticket we're looking at what's causing a lot of the pain out
43:47 there this is basically us looking at any abnormalities in multicast broadcast
43:53 relative to uh what is out there seems very simple but if you think about it at
43:58 the scale of missed millions and millions of APs deployed and switches out there every board every minute the
44:06 cloud is watching for abnormal traffic non-trivial problem no one in the
44:11 industry is actually looking at traffic at the level where we're looking at and running anomaly detection every user
44:18 every minute every port every minute it takes a real Cloud to be able to do this
44:24 in the spirit of moving uh forward Bob talked about and Jeff Aaron talked about Marvis client is a big deal for us
44:30 Marvis client is actually uh uh our SDK we've had a marvelous client SDK for
44:36 Windows and Android one of our large customers you know uh thousands and thousands of android devices that are
44:42 deployed with Marvis land out there today we're proud to launch the Mac SDK so uh so essentially uh uh Marvis Klein
44:50 you know um if you if you are an Enterprise that issue macro Pros to uh
44:55 to your employees you could push out this Marvis client out there and get additional data very interesting
45:02 additional data just removing some steps right this is uh you know something that you have to manually put together on hey
45:08 what was the driver version radio firmware version of that device you know simple stuff that you can know if you
45:14 push this out there boom it's Marvis is always learning and collecting this data
45:19 right where this thing becomes super powerful is when you take this user and
45:25 say okay I have a marvelous line uh on this user and and you know that Marvis
45:30 client is streaming data now put that in the context of hey I see I know what the
45:37 AP is seeing from an RSSI perspective what's the client view of the RSSI we're talking about real clients actual users
45:45 actual impacted users being able to put them side by side and see uh you know
45:50 the data from the client and the data from the AP now let's take this to in my view one of the coolest Renditions in
45:57 the in the midst uh conversational assistant in Marvis is is seeing the roaming picture of users right and so
46:04 put uh put the marvelous client on a MacBook Pro you not only see the roaming picture from the uh the access point
46:11 side you know what the AP sees but more importantly the client-side view of the
46:16 RSSI every time a device is roaming right super awesome being able to see all of this put together side by side uh
46:24 now with mac and windows obviously iOS has their restrictions hence there isn't an iOS agent per se but Android uh um
46:31 you know Windows and Mac where we're open for our customers to start to try this right super cool
46:37 now uh the the the next piece I want to go to is uh Marvis as a member of your
46:43 team uh this is uh another uh really cool Edition where you don't have to
46:49 actually log into uh uh sort of um you know Marvis and and the Miss dashboard
46:55 but what if Marvis is an app on teams right that you could add and literally
47:01 add Marvis as a member of your team's channel right and so we're introducing Marvis as a member of your team's
47:08 Channel and so what you could ask The Marvelous conversation assistant you can now natively ask in teams literally at
47:14 Marvis you know yes please so so that means that um people that I don't trust with dashboard
47:22 access can still ask Marvis questions through teams which we all use already
47:27 anyway if if you add them to a team that that you have access added Marvis to if
47:34 let's say someone else let's say help desk you know with their own API token with their own access you know has
47:40 access to two sites and and uh and you know only Observer access that's all they'll see right but they can still add
47:46 Marvis as a member off but we we can add it to a team and add invite users invite users to it yeah they can talk to Marvis
47:53 that's correct without ever ever having to worry about getting access absolutely absolutely wow absolutely yeah yeah
47:59 um uh uh you could basically um say uh This Is A team's channel
48:05 locally and I'm just saying Marvis you know what's going on and this is a team's Channel with me and my colleague
48:11 here and and we're talking to Marvis together uh based on me adding Marvis uh
48:16 into that themes group right so really powerful stuff and um uh with that I'm
48:23 gonna just finish off with the impact Marvis has in the real world and uh so
48:29 I'm gonna rapid fire through this we deployed Marvis at a retail store they
48:35 have a four retail store Network 4 000 retail stores um 1.5 million ethernet ports being
48:43 watched by Marvis out of 1.5 million ethernet ports I'll leave this goes back
48:48 to 400 of them were constantly flapping they were flapping for years you know
48:55 causing DHCP you know every time they reboot they get DHCP connect radius reboot get the hcp connect radius reboot
49:02 constantly you know 1.5 million ports 400 ports constantly flapping they had
49:09 no idea happening for years with their previous vendor the moment Marvis and wired Assurance came in we were able to
49:16 show visibility in a very large University in the Northeast Marvis showed them capacity constraints right
49:21 being able to out of 10 000 AP deployment we went we sent people because Marvis said you have a capacity
49:28 issue we sent people to seven places right 10 000 deployment a very large
49:33 technology manufacturer they said no I don't trust this I don't believe Marvis can detect bad cables you know they had
49:39 Juniper switches 100 switches in a factory running for 800 days we said
49:44 okay you know if this they said if Marvis is this hot shot you know I have Juniper Network right now let's go try
49:51 this literally without rebooting the switches upgrading code none of that just connecting it to the Mist Cloud
49:57 those 100 switches four out of you know 100 switches is 5000 ports let's say 4 800 100 ports
50:05 four out of 4800 ports had a bad cable they walked that cable all the way to the end and the user said for the last
50:12 three years my Wi-Fi was more reliable than my wired ethernet right this user had a bad cable for four years they
50:18 didn't know right so Marvis truly is impacting user experience this one is a
50:23 favorite one for me a very large Financial Association at top five financial institution in the world he's
50:29 migrating 60 000 access points from from their existing vendor to mist in the
50:34 very first deployment Marvis saw a radius outage that prevented a massive
50:40 outage for them on the on the radio side uh so on and on uh very interesting stories Wes is going to go through some
50:46 of these as well but um that's the Marvis section um hopefully you saw a lot of cool new
50:53 innovation and we're just scratching the surface