AIOps for Networks: The Impact of AI on the Future of IT Networking Operations

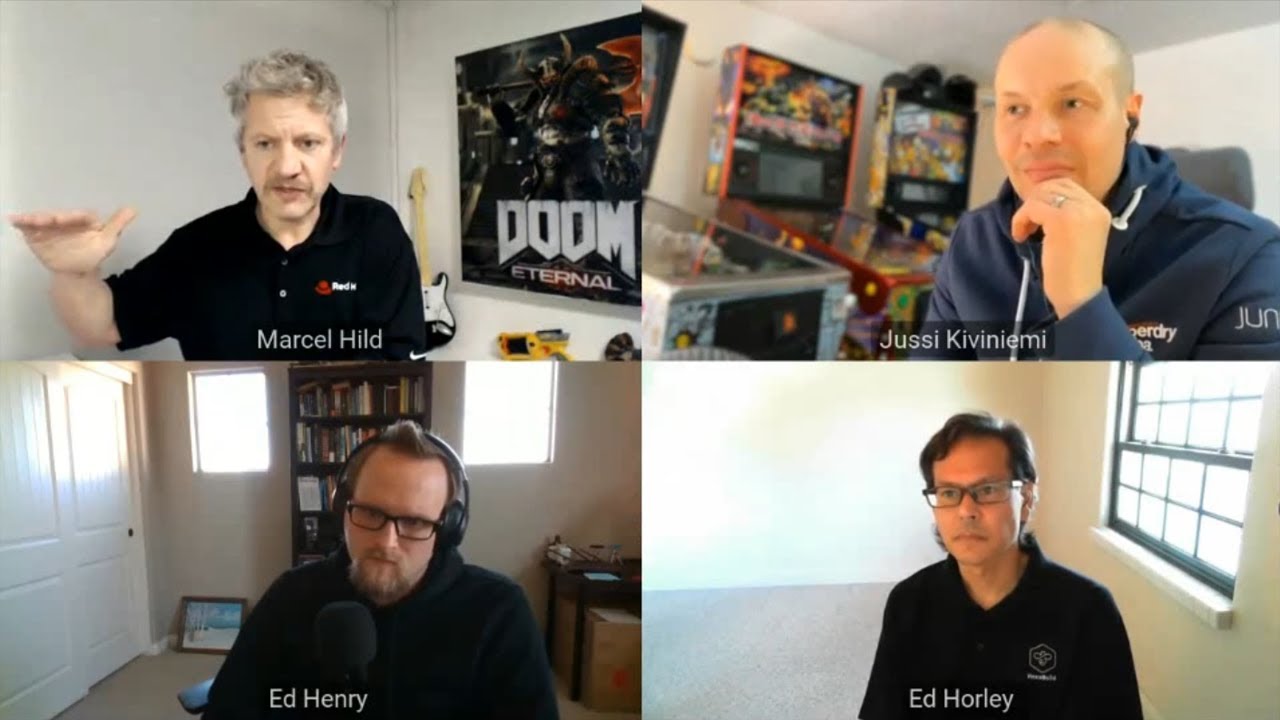
How to seize this opportunity to strengthen network operations.
Artificial Intelligence for IT networking operations, or AIOps, is finally here. But how will AIOps impact the daily lives of network engineers and IT networking practitioners? Find answers from this roundtable discussion featuring experts from four different organizations. Tip: Watch the demo of Juniper's Marvis Virtual Network Assistant.
2:56 Will AI take away my job?
8:00 What is AI? How is machine learning different from traditional programming?
12:25 What are forms of intelligence?
16:00 Understanding and assessing AI systems
18:22 Understanding data, human priors, and representations
21:30 Training AI
27:00 Applying learnings from computer vision to networking
28:00 A grand theory of networking
30:45 Breaking with traditional networking approaches
32:00 Modeling the network
33:00 Demo of Juniper's Marvis Virtual Network Assistant
34:10 Other tangible examples of AIOps
37:25 Starting the journey of AIOps
41:45 Building a data sciences practice
55:55 Recommendations for resources
1:02:00 Where do we go next with AIOps?
You’ll learn
What is AI, and how machine learning differs from traditional programming
How to apply lessons from computer vision to networking
Resource recommendations, and what’s on the near horizon
Who is this for?
Host

Guest speakers



Transcript
0:00 hello good morning good evening good afternoon everybody and thank you for joining the ai ops in networking panel
0:08 discussion um my name is yusi and i'm truly honored uh to be joined by this
0:15 distinguished guests uh today so today we're gonna
0:20 talk about ai uh machine learning and its impact uh especially to to our
0:27 networking uh the network engineers of us the network managers
0:32 so without further ado let's do a round of introductions of our of our panelists
0:38 ed horly why don't you start oh hi everyone
0:44 uh ed worley i'm the ceo co-founder of hexa build and uh we really focus on on
0:49 ipv6 as a transition but obviously automation is is key network automation is key for what we're doing because we
0:56 think that's the direction cloud networking iot is the direction that things are going and that's obviously an area of
1:02 impact for ipv6 and things around ai machine learning seem to be cropping
1:08 up more and more around around those subject areas so it's super important for us to sort of have a good understanding of what's happening there
1:14 so that's that's me been doing i.t and operations for 20 plus years
1:19 thank you for joining ed uh then uh so so that we have enough confusion for it
1:26 for the host of the panel we have another ed uh ed henry uh how are you man thank you for joining
1:32 i'm doing good man thanks for having me uh ed henry i work in the office of the cti or the ctio office at dell
1:38 technologies and i work on anything and everything machine learning related that can span
1:43 the gamut from robotics and computer vision through to infrastructure automation like this conversation will
1:48 be about i'm happy to be here excited to talk a little bit about where this kind of
1:53 technology is headed thanks for having me man of course thank you so much for coming
1:59 and uh marcel healed uh good guten habens begets yeah good good numbed i'm good
2:07 i'm i'm fine so it's 6 p.m here out of germany and i'm also
2:13 working in the office of the cto this time red hat so one layer up the stack and we try to make sure that ai as a
2:20 workload works great on our platforms but also i'm looking specifically into
2:27 how can we use all the metrics and locks being produced by all those machines to
2:32 push forward to that vision of the self cluster somehow and i've been doing that
2:37 for the last three years and i'm happy to share my challenges concerns and what lays ahead
2:46 sounds good thank you so much marcel for joining as well uh so so speak about challenges uh let's just
2:54 start with the elephant in the room so uh is is this gonna be happening like um am
3:01 i gonna be losing my job is my job going to drastically change as a network engineer as an as an i.t ops person
3:08 how's this all going to pan out what what do you guys think
3:15 yes why don't you of course of course you will lose your job at
3:21 least i mean your job will be different than it was before it's it's pretty much like um people were riding
3:29 horses caps a century ago but we still have people riding caps or
3:37 driving caps and the same will be true for operation cycle you won't be the person um
3:44 i don't know um like doing your job as you did it before but
3:50 you will be uh assisted by some some tooling somehow so i think it will change a little bit but you certainly
3:56 will not lose your job i think we will need more humans guiding those
4:02 in the end we we need to train them somehow
4:08 absolutely and and ed uh eduardly what are some of the examples like what
4:15 kind of jobs are are on the line first uh and especially if we focus on the on
4:20 the i.t side of things or or outside of or outside of it what do you think
4:26 i think i think it's i don't think there's going to be jobs on the line i think the the definition of what your role is as a job is going to change to
4:33 marcel's point it's it's going to be that you're going to focus on the things that are actually of high impact within
4:40 the business because we can take a set of of chores and duties that maybe were very repetitive
4:45 maybe a little grinding a little a little um you know something something that could
4:51 be stamped out in a more automated way and and we're going to shift those sets of workloads off onto computer systems
4:58 as opposed to something that we have to run and maintain and build you know a set of workload and flow and yeah this
5:03 is a great great slide from from jason edelman talking about that our behaviors
5:09 really haven't changed for you know for well over a decade maybe even two decades in terms of how we we do
5:14 specifically in networking how we do operations and i think we're starting to see the evolution of of investments by
5:21 the major you know network players to really change how we think about building deploying and operating
5:27 networks and and to this point maybe we can have a quick conversation about about how automation's really changing
5:33 that and then how ai is actually changing that portion so i i someone you know tell that to ssh isn't
5:39 a big elevation and maybe in security but that's about it so i think i think the points well taken
5:45 there very good uh it henry is there like
5:50 some other like fears or misconceptions around ai besides this uh you know i'm
5:55 gonna lose my job tomorrow any any other that come to mind um
6:01 nothing specific but i guess in the well there's a lot but in the realm of like losing your job people should
6:08 probably understand that machine learning is really just kind of a more robust way to automate
6:14 and robust being a function of situations generalization across situations in which somebody needs to
6:20 make a decision right and the reason why this technology is becoming pretty popular is because
6:26 humans exist on the perceptual plane right like we we see the world we hear the world we interact with the world
6:32 through our perceptual processes and we interact with the systems that we create through those perceptual processes so
6:38 you see a lot of advances in the perceptual space of machine learning uh headed toward being applied to the
6:45 way that we perceive our systems like we see today right um so i wouldn't necessarily be a i do
6:52 like the idea of you know what marcel and ed had outlined in that your job will be different
6:57 but it's just yet another layer of automation and it's kind of needed it's you know the
7:03 amount of connections uh with respect to devices that exist uh on the internet today is is a function of
7:10 of the amount that humans can support today right so in order for us to be able to kind of grow that number of connected devices we need to think about
7:17 how we do this thing called management a little bit differently yeah and our our jobs have been evolving
7:24 like obviously for centuries anyway with tractors and you know then computers and and what
7:30 have you so so this isn't that much of a dramatic shift uh at the best and and
7:36 very likely is the shift from kind of like for network operators for example like i think from firefighter more to an
7:43 architect type of a role and like you said from repetition into like more more interesting tasks
7:50 then if we if we quickly uh then move from the big elephant in the room into
7:56 like a little bit defining what is ai and machine learning and and
8:01 you know how is machine learning different from a traditional computer uh program how is the machine learning
8:08 program different anybody want to take a quick stab at uh kind of clearing that out
8:14 yeah i can jump on that one if you'd like initially and then everybody else can throw something out there as well um so
8:21 i tend to not be categorical in thinking with respect to what is artificial intelligence
8:28 i don't think we know what intelligence is yet sufficiently enough to even wrap a definition around it but we do know
8:34 what you know learning roughly learning looks like so from a machine learning perspective it's this idea of being able to generalize
8:41 right so given an input into the system can i make a decision effectively in
8:46 understanding what that input is or is not or what it should or should not be or have i have i seen this thing before
8:53 or not so if we were to liken this to say something in the computer vision realm uh given all of these pixels that i see
9:00 in this particular image what is in this image right if i were to imagine writing an if-then
9:05 statement for exactly right if i were to imagine writing an if-then statement for all of the images of dogs and muffins or
9:13 chihuahuas and muffins like in this example that would be infinitely long given the
9:18 input dimensionality of the particular image right more traditional programming is inverse
9:24 to that where i would write all of those if then statements i would develop an algorithm to be able to solve this particular problem this concept of
9:30 generalization and machine learning can kind of be interpreted as finding that algorithm as opposed to encoding it by
9:37 hand [Music]
9:42 data is becoming also part of the program so before you would have
9:48 your fixed set of inputs data then you would write your program which is bound to that inputs
9:54 data and then you would have run it and obviously you would have ci tooling and gating mechanisms to make
10:00 sure that your program is still valid depending on the data that you designed your program to
10:07 now with machine learning since the program itself is so much based on the
10:12 data the data itself is also becoming part of the program so you will have to rethink how you would put
10:19 a program into production because if your data changes you would ultimately also have to adjust
10:25 how your model or how your deployed machine learning thing
10:31 is is is acting so things like data drift are becoming more and more
10:36 important than in the previous world where you have had input machine output
10:44 and that also makes uh qa for example uh a bit more challenging right right you
10:50 don't have a linear right answer anymore every time that the right answer will always stay the same am i right
10:59 yeah i think i think i think that assessment's good i think the other part at least for me when i when people talk
11:04 about ai in general terms i just try and correlate that to let's try and mimic something around
11:09 human behavior right and that's a wide breadth of of topic within itself but i think the attempt to
11:17 try and make computers mimic any sort of of of related human behavior
11:22 and humans are pretty adaptable looking at different data sets and building correlations between them we're good at looking at you know
11:29 taking different modalities of data right we can take audio data and visual data and put correlations together between them which
11:35 is a very unusual thing to do and so teaching computers how to do that and mimic that sets of
11:41 behaviors i think it's sort of what as a general bucket i can sort of i sort of consider ai and then machine learning is
11:47 what the sets of datas and algorithms you can you can place in there and i don't know if that's accurate or not but that's sort of how i look at the world
11:54 i'm interested to hear what ed henry has to say about whether i'm accurate on that or not but but that's sort of how i
11:59 see the general journal bucket i think that that's that's actually also the powerful thing right so not not just
12:07 that we humans are really adaptable and prone
12:22 so i'll jump in it looks like maybe marcel's having some connectivity issues um when i mentioned earlier i wouldn't
12:28 necessarily be categorical about this idea of intelligence um what i mean is we tend to use ourselves as a ruler kind
12:34 of arrogantly but we have forms of intelligence that exist all around us right like in animals and even in
12:40 patterns that aim on the animals seem to exhibit like flocking patterns and birds and things along those lines right so i
12:46 agree um but from the perspective of like creating a larger general intelligence i i'm not convinced we
12:53 understand enough about what that even means yet to head down that path so at least we can
13:01 we can agree on the like uh ai something probably adaptive and autonomous at
13:07 least sorry sorry go ahead well and and that's why i put it in the
13:12 category of sort of behavior maybe human behavior isn't the right thing to ed's point that there's animal species
13:17 there's you know bacteria there's other things that have a sets of behaviors that definitely are
13:23 you know could be argued have some sort of look like intelligence
13:28 that's a general bucket to put it in but that's why i say it's a set of behaviors that we're able to discreet around and i
13:34 think that's the important thing is that that's to me is the general bucket and then you start getting into as a slide
13:39 showing you know you know if you if you use that then you can start breaking down what you sort of think about around the data science the deep learning the
13:46 machine learning the the other areas that sort of fit into that category but i think they're all there their own goal
13:51 is to try and mimic our sets of behaviors right our sets of of outputs about how we interpret things
13:57 we're trying to rationalize that with computers that can do something similar to what we're up to it doesn't mean
14:02 they're right all the time and it doesn't mean we're right all the time either right but i think but i
14:08 think it's important that that's what we're attempting to emulate within the within the environment so it's that's
14:13 what we're trying to gain insight around so i think if if you take that viewpoint then you sort of get to what the goal is
14:18 which is the goal is we're seeing a set of behaviors and as humans we normally do x maybe it makes more sense to do y
14:25 here's a here's an example of how that's a better output or you know we normally do this and in
14:30 itops this is a really good example security breaches and how do you detect anomalies within your network
14:36 and to ed henry's earlier point when you have so many devices it becomes so large it's very simple when you have a discrete small number that you can
14:42 measure on two hands right like i got 10 devices it's pretty easy for me to look through the logs i probably know all the ip addresses for all those device sets
14:49 and then you start putting a lot of extra zeros after that for the total number of devices it becomes a
14:54 much bigger just data ingestion problems so that's the big data side and then what's interesting out of that data
15:00 becomes a much bigger problem and so that's that's where computers can really help us to get better insights and
15:06 bubble that information up now are you having a human still make decisions around that
15:13 well sure that probably makes the most sense but you want a fast way to bubble that data up to them and that's my
15:19 specific example around networking i mean there's obviously tons of other examples in other areas but i think those are some of the things that are
15:25 very that are changing in the landscape for folks in terms of how they operate today yeah and i'll agree with ed um
15:33 i hope you can near me again sorry to interrupt you oh you're back yeah i think the whole european on 24
15:40 went down for a bit but many people doing homeschooling here
15:49 i hope it's homeschooling so sorry ed ed henry you were uh
15:56 horribly we're about to say uh yeah no i i think i think this is the point at henry's original point around
16:03 around sort of that behavior side it's it's what's the interesting sets of data that in that indicate a set of behaviors
16:09 that are important for for folks and how do you bubble that up when the data sets get bigger and bigger
16:14 and bigger and i think the challenges for us as operators is traditionally we've run relatively discrete smaller
16:21 you know networks and systems and as you start looking into what the cloud operators are doing when you start looking at what larger fortune
16:28 enterprises are doing it it's not sustainable to try and hire that many people to run that big of an
16:34 organization and get enough value out of it but you still have risks that are associated with it so you want to you
16:40 want to be able to prioritize and and provide the greatest value for you know risk mitigation and that's
16:47 things around security that's things around misconfiguration that's things around you know just adaptability of
16:53 being able to solve business problems and making sure your application teams can deliver what they need to and those those operation principles at scale
17:01 become very hard problems to solve and this is really the area where i think you're starting to see a lot of value being added to the
17:07 ecosystem about saying how do we you know at henry's earlier point how do we make sure that
17:13 automation is delivering what we expect that we're getting the outcomes that we expect and that we're getting the consistency and the supportability and
17:20 all the other things that are important for wine and business owners right and no one cares if your business can't run
17:26 because you can't get your app to run no one cares about how well your system is actually behaving at any given point in
17:31 time because it doesn't solve your customer problem and so understanding those systems and making sure that stuff
17:36 is taken care of on the underlay is really important and that's where i think this first set of ai ops is really
17:42 making a difference for people because that means that a small investment in a team that's well
17:48 well built and understands how to use these tools is going to outperform a much larger team who does not have the
17:54 insights and availability of these tooling sets to do the same work at least that's that's what it appears
18:00 to be in terms of what we're seeing from the cloud operators versus like the judicial folks building infrastructure
18:06 sure and and when you align you know a lot of what ed just said back to you know what is machine learning and we can even talk
18:12 about from the like it and ai op space or whatever moniker acronym you want to assign to it
18:17 when we head down the path of applying machine learning a lot of the time for the first thing people reach for
18:22 are things off the shelf out of the perceptual realm right so we look at like you had a slide up a minute ago on
18:29 deep neural networks right like the the advances that have been made there are interesting in the perceptual space and
18:34 they're starting to be uh applied as kind of like a tool in other areas of science but the important thing to
18:40 capture there in understanding how or why is this working and why now
18:45 is that we're capturing human priors in the data sets that we're using to train these models so to ed's point and what
18:51 does capturing a human prior mean right so in the realm of like it ops it's understanding
18:56 how you have this human that's been running this giant system of devices that has integrated all of this
19:02 information over many different time steps right so inside of our heads we all have maybe a rough diagram
19:09 of what this particular piece of infrastructure looks like how it's interconnected all of the relationships that exist between the different
19:14 components of this system right decomposing that into a representation
19:20 that works for machine learning is a tremendously hard problem and the reason why i say that is because we have no way
19:26 for me to kind of download that internal representation that you have inside of your head so what do we do we have to talk to
19:33 humans in order to understand how they're even building this internal representation of their head and then understand how they're using the outputs
19:39 of that system to build that internal representation right whether it be configuration information or whether it
19:45 be operational statistics or whether it be you know the fact that somebody in an executive board room said that that's
19:51 the way i want it to look and i'll go do it the reality becomes when we apply machine learning to these
19:58 problems it's incredibly important that we figure out what it means to capture those human priors
20:03 in a way that is sufficiently representative of enough of the problem we're trying to solve
20:09 and you can do that in a lot of different ways deep neural networks is one way but one of the other things that i think the academic side of the world
20:15 and the realm of machine learning and artificial intelligence generally has started to realize is deep learning still doesn't solve a lot of the
20:21 fundamental problems that exist and what i mean by that is like you guys had mentioned earlier the world changes around us right you have this
20:28 distributional shift that happens constantly you and i get on an airplane six hours later we're in another part of the country the world looks entirely
20:34 different right or at least in jesse and marcel's side where you're in a whole different country right
20:40 but the world looks entirely different that's a distribution shift on the pixels or at least the inputs photons
20:45 that your eyes are receiving right that out of distribution generalization is an unsolved problem so we have to contin
20:51 this is the argument that i make a lot of time on will your job go away as an operator i don't think so because we constantly have to query you we
20:57 constantly have to ask you what should we do in this particular situation now can we capture a sufficient representation enough to run
21:03 infrastructure maybe i don't know but in the world of machine learning things are empirically driven i need to be able to
21:09 go and prove that empirically to determine whether or not my model performs better than you as a human
21:14 right so i'll stop ranting now but anyway i'll open the floor for anybody else who wants to jump in
21:21 yeah that's that's really really true and when it comes to like the model and how how the ml has
21:28 been trained uh it all comes down to like how widely it has been trained right like as as in this picture for
21:34 example um you know this guy puts his uh wi-fi access points in the freezer and
21:40 in the dumpster to train the extreme situation so let's talk about that uh training and and data uh for for a while
21:47 so so um how important is is like an unbiased data set in training and what
21:54 what kind of extremes do you need to account for and on the other hand uh like what kind of extremes do you need
22:00 to avoid with the data what kind of caveats i guess i'm asking is there with training and the type and amount of data
22:08 there there is we know that you know the better the data is uh the better the ai is and if there's you know crappy data
22:14 then the ai also is very flawed what are your thoughts on that
22:20 yeah so i think that that's that's true especially with the bias data so if you have some
22:27 data that is just full of lies then you can only treat a model that is producing
22:35 lies but i mean that's super super important when it comes to
22:41 decisions where actual human life is impacted such as models that
22:50 may guide a police police thing
22:56 or a um for in jurisdiction right um so that that's super super critical and we
23:01 and there are a lot of research going on with regards to detecting bias in in models or when it
23:08 comes to face detection or something like this so we all saw these um things where where the model is um
23:16 really bad at detecting black faces because it's trained on primarily um white faces
23:22 but when it comes to i.t data and i think that's what more of the folks here are uh concerned with whether your
23:30 machine is being rebooted because the model thinks it's a bad machine there's nothing really there's not such a real
23:36 impact right so that's that's completely okay i think here it's more towards do
23:42 we have to train model every time on every data center
23:47 and learn from scratch again and over and over and over again because i that that's that's what's currently happening
23:54 in most of the tools that are applied to i.t problems so i can buy something off
24:00 the shelf that gives me um linear regression or um anomaly detection or
24:06 event correlation but i always have to train it on my data set so i i haven't
24:11 heard of a product i mean there are probably some products there but um like a
24:17 kubernetes cluster that ships with pre-trained models on the um on the on the outages that are usually
24:24 seen with that cluster if i step even one step back and say a database let's
24:29 let's imagine postgresql which ship with models that detect misconfiguration or
24:35 detect outages before they actually happen because we
24:40 trained some baseline models on data that has been running from the
24:47 community or from from from other folks so i think that's that's also an important aspect when it comes to data
24:54 because ultimately what you're saying is true a model can only be so good as the data as it's been trained on
25:02 so uh then those that have uh access to most
25:08 of the data for example in networking will have a huge advantage it will not only be their existing software and the
25:15 domain experts there but also you know data will play
25:20 like obviously a bigger and bigger role uh in companies having a competitive advantage
25:26 and and then like what are the efforts in democratizing uh all of this data today these kind of events are like uh
25:34 these kind of initiatives are happening but they seem to be pretty small compared to you know these closed uh
25:40 corporations that's just harvesting tons of data
25:46 so i'll jump in a little bit um from the machine learning side of the world uh and again in the research side
25:53 of everything i mean arguably a lot of the uh advances that
25:58 were made were kind of powered by the data sets that were were collected so when you look at
26:04 things like the imagenet datasets a couple tens of millions of images that are labeled right
26:11 that data set was key to kind of unlocking the i guess
26:17 old approaches for some value of ahold in in the world of machine learning and
26:22 scaling them to be superhuman for some value of superhuman and the perspective of performance right
26:29 when it comes to networking and infrastructure in general those data sets frankly they don't exist
26:35 they don't exist in the open science space they don't exist in the open source space so i think that's why we see
26:42 a lot of kind of just firing from the hip in the world of machine learning and seeing
26:47 what works i think what will happen over the next probably five to ten years i don't know i'm probably going to get shot for
26:54 throwing an actual number out there but over the next n amount of years right um we will probably start to realize that
27:00 in the world of networking we have to do a lot of things much similar to other
27:05 fields like computer vision or natural language processing or speech synthesis or or the perceptual realms generally if
27:12 there are problems that we are trying to solve figuring out how to collect a data set that represents that problem is
27:18 imperative for this whole process to kind of take off and work right um so i think the other thing that's
27:24 kind of lacking there especially in the world of networks is there's no like kind of grand theory of networking and
27:30 what i mean by that is in the world of say computer vision we have a rough approximation of how like your retina
27:36 works and we've baked that into things like convnets right convolutional neural networks um the idea of these
27:41 convolutional kernels that your filters that exist inside of the layers of the network are a rough approximation to how
27:47 your retinal works and actually proceeding back into the visual cortices of your brain but the idea is we don't necessarily have a
27:54 great [Music] grand theory of networking yet i think there are a lot of people who
27:59 are headed down the path of what does this look like if we were to take like graph theory and apply it to infrastructure generally
28:05 but again a lot of the progress that we all hope for will come from open source and open science i think that that's
28:12 really kind of the only way forward and when it comes to larger organizations you may see blog posts and research
28:18 papers published and generally ideas that are thrown out there that
28:24 have some sort of state-of-the-art performance on a given problem you'll notice the one thing they don't publish is their data set
28:29 and i think we even talked about this in our pre-meet up right the idea is that data set actually
28:34 captures the representation of the problem you're trying to solve and it's your edge right it's how you are able to
28:39 differentiate with respect to what potential problem or or methodology in
28:44 the world of machine learning can apply to this problem right yeah i think i think there's some big
28:51 changes or evolution change that's happened in the last you know five years or so that's changed the
28:56 industry in terms of capabilities to be able to do this before you had to write on-premise software that did to marcel's
29:03 point did all the data aggregation collected all the data and then tried to provide some insights based off of your limited data set of how you operate your
29:10 network and hopefully your network was big enough something interesting was actually happening that provided some multiplier effect to
29:16 sort of reduce workload or gate or provide the the ops team some better insights about what's going on versus
29:22 what they already knew the change today is that with cloud first principles around networking
29:28 technologies and being able to aggregate that in cloud and having cloud operations happen and streaming and providing that common
29:34 data set across thousands and thousands of customers you're able to gain a set of data
29:39 insight and to at henry's point yes it's it's that specific vendors capability to
29:45 provide insight but they're getting a much bigger aggregate of data and suddenly they're able to provide better insights for those discreet customers
29:52 they're pushing that insight into their capabilities of their products to provide that insight but the community
29:58 overall is is able to then take advantage of those insights and capabilities that they're building into the product based off of a larger data
30:04 set now do we all need to open up all data sets in order to make push things forward
30:10 there's you know the altruistic side of saying like yes that should be where the industry tries to strive for
30:16 but as we've seen with the ietf and as we've seen you know the sort of the the collaboration side of networking we're
30:22 sort of vacillating between all opened all closed all open and and there's got to be a happy medium to allow companies
30:29 and entities to exist and have a product to build that and also the data sets that provide greater insight i think the
30:35 funny part is is that we've codified everything in at least today in in standard sort of config management tools so to marcel's
30:42 point earlier right the way that we define things and the way we find the human learning is just to say like we don't understand
30:48 what's going on with that compute unit just blow it away and restamp it because that's the easy thing to do um that may
30:54 or may not necessarily be the answer that's appropriate for that particular device but it certainly is a way to
31:00 solve the problem for how we deal with things today maybe with better insight later on we'll understand how to tweak systems to to to
31:07 make that better but that's the quick and easy way that we could to find that right you run your ansible playbook
31:14 things back down again and say get rid of that system uh let's get the behavior that we turn it on and off again
31:20 yeah well yeah not quite the you know not quite as but you know have you turned it on turn around again yeah but
31:25 i think i think what it is is we see a set of behaviors that we don't like it's just go replace the thing so it doesn't
31:31 do the bad behavior anymore as opposed to understanding where the behavior sets come from and i think that's the next
31:36 innovations that we're trying to get to is understanding why maybe the network is doing what it's doing as opposed to
31:42 saying like we just don't want to see that sets of behavior so let's just go fix those sets of behaviors
31:47 all right so there is a connection yeah go ahead it's uh no no go ahead uh mr
31:54 henry oh i was just gonna say and that that brings me to you know the representation of the network right
32:01 like we have protocols that exist like syslog we have protocols that exist like snmp we have protocols that exist that
32:09 allow us to query the current operational and configuration state of infrastructure generally right
32:14 but i'm not convinced from the machine learning side of the world that that's the right representation and what i mean by that is like that's the right input
32:20 to said machine learning model i think what we're going to learn over the next couple of years as well is that we can probably start to
32:27 rethink how we present that information because when you look at things like syslog and snmp and all these other
32:32 protocols and even if you head down the path and networking things like netconf and yang it's a representation that's
32:38 meaningful and useful as input to the perceptual system of a human right
32:43 yeah where in reality we could probably come up with different representations that are more meaningful for some value of meaningful for capturing information
32:49 with respect to the underlying system that we're measuring i.e networks or infrastructure
32:55 absolutely absolutely and and if we uh that's actually a very really good point and while while you were talking i
33:01 apologize for missing the faces but i was showing some of the things that uh juniper mist for example is is doing in
33:08 this space so uh just as an example we have uh conversational interfaces we we have a
33:15 query language and but and and kind of this like google type of networking thing where
33:21 you know you can ask how how is ed henry doing and then it will kind of break down your connectivity into parts and
33:28 say say is there significant problems where are they and you know what are they correlating with and all that kind of
33:34 stuff uh and then there's the automatic kind of analysis of pro problems identification and analysis of problems
33:41 what do you guys today see as as some of the tangible examples of ai uh where
33:47 where you know what people should at least be doing or email uh in networking i mean
33:53 uh what where can people where are the low-hanging fruits uh for i.t or networking people uh
34:02 you know what are some of the tangible examples for you guys
34:08 i think i can show you so i've been looking a lot into time series metrics
34:13 since i'm working mostly on kubernetes clusters and if you install a kubernetes cluster you will end up with um 10 000
34:20 to 2000 time series um at least and it's super super tough to come up
34:28 with meaningful thresholds um in yeah getting getting on top of all these
34:35 time series i mean you can you can hard code things but
34:41 at just looking at the vast amount and using some of the
34:46 exploratory data analysis tools that data scientists have like
34:52 just plot all the 1000 time series and find out which are correlating which
34:57 have the most fluctuation which have common labels that's that's basic that's
35:03 the hello world of an alpha data size and using those tools and point them at your data set i think
35:10 that's that's the first thing that you can do and that's something that you can easily do within a month so you
35:17 spin up youtube just look at how jupyter notebooks work and then how you deal
35:22 with time series and then you have prometheus collecting all these time series and then connect them that should
35:28 be pretty straightforward engineering task and then the then the next step would be let's take
35:34 one really simple time series that i'm failing to come up with sensible
35:39 thresholds and just do some linear regression or some more advanced
35:44 prediction stuff so that i can be notified on anomalies because normally
35:51 in the end it's nothing else then this thing deviates from the predicted
35:56 value and even if you look at prometheus it has um it has two built-in
36:03 tools or functions to do a projection into the future i think
36:09 it's a linear regression and the other one i'm i'm blanking on now but even
36:14 applying these i think that's that's super low hanging fruit and that that will get you started with um applying
36:19 the aiml tooling to your domain
36:28 definitely a good uh good starting point what about one of the ads uh any any
36:34 you know examples of uh how other examples of how a network engineer
36:39 uh besides purchasing juniper uh networks or or switching or or wi-fi and
36:45 any other uh good places to start and what marcel was saying is is like uh
36:52 also requires some some of course you know uh development skills some some data science skills as well uh is there
36:58 any where is the lowest hanging fruit where should people get started on the ai journey anyway uh especially networking
37:05 people i mean let's say you know you already know some scripting and some python what's next
37:13 probably for me on the opera just because i deal with a lot of customers in the in sort of the fortune 500 and
37:18 the operator role so so my ben's a little bit there so i'll just put that out there as a caveat i think many
37:25 people are starting down this journey around logging today already so the logging data that they're trying to get better insight around i think most of
37:32 the major networking manufacturers are providing solutions around how to get better data insights out of that so that's a great super easy lift start
37:39 location for them to go the other funny one is that i i i'm amazed how many network engineers are
37:45 unwilling to get out of their seat wander down the hallway go talk to their app dev folks who probably already have
37:50 prometheus kafka and a ton of other uh complex uh data ingestion systems
37:56 already in place and say like can i ship you a portion of my log files and can we start playing around can you start
38:01 helping me to look into the data sets that i already have that i'm producing it's
38:06 really good can you guys take a portion of that i don't need long-term storage i just want you guys to start showing me how you guys are using this for the
38:13 business that we're running today can you show me how to use and start maintaining and running some of these tools that are already existing probably
38:19 running within your environment and that's the fascinating part for me is it's just the lack of communication the lack of of being willing to explore
38:26 and wander down the hallway to the probably a team that is doing this sort of work and saying can you guys provide
38:32 me some some baseline some tooling some collaboration together so that i can get some some information even out of our
38:37 own systems they may tell you to go run away or go read this book but that's okay go do it and then come back to them
38:43 after you invest a little bit of time to understand what they're dealing with
38:48 you can do the reverse to them you can say you should invest a little bit of under time understanding what impacts you guys are having on my network
38:54 because that's a legitimate conversation too but it does allow you to have a much better collaborative conversation with the folks that are actually trying to
39:00 solve a bunch of these problems within major organizations it's something that you really should be uh
39:06 involved with i don't know you know ed henry if anyone's actually approached you in that method of saying like hey we'd like to get better data
39:12 insights in our operation side never mind what you guys are trying to solve from the business angle side but what better what better things can we have in
39:19 our op side to give us that capability i don't know if that's been an experience you've had or not
39:25 yeah it is uh and interestingly enough the operations usually maps back to money somehow so people are usually interested
39:32 in figuring out how they can enhance that experience um i would approach that question of like how can people get
39:37 involved from a few different angles i think the first angle i would come at would be you know i outlined earlier that as somebody who's working in
39:43 research and engineering in the world of machine learning i'm trying to capture the prior that is you as a person right
39:49 i'm trying to capture the prior that is the internal representation that you have of what your infrastructure is and what good or bad means with respect to
39:55 that infrastructure so just start to think about the questions that you would like answered
40:01 start to think about the problems that you approach every day that are pain points that you haven't been able to
40:07 quite automate around yet um and then second would or at least two of n would be there are ways that we've kind of
40:14 rallied around this in the world of infrastructure and networking in the past i know there's a repository if it still exists or not i don't know on the
40:19 internet of pcap files where everybody was just posting representations of say protocol exchanges inside of pcapp files
40:26 so people can start to download who are interested those pcap files and understand what the connection semantics
40:31 for that particular protocol looks like right the same thing could potentially apply in the world of infrastructure maybe
40:37 don't give us a capture of your your specific production infrastructure but if you have something that looks and
40:43 feels a little bit like it from a lab perspective you know that this particular infrastructure exhibits these
40:48 particular properties of this problem that it is that you have feel free to publish that right that's an example of an open data set
40:54 aligned with a particular problem it is that you're trying to solve and then lastly i will highlight exactly
41:00 what ed said as somebody who practices in this space the first thing that i do when somebody asks me a question around
41:05 can you solve this problem with data science or machine learning or whatever it is that you want to call it i find out the people i try to find the people
41:11 who are the ones that deal with the problem every day because they're the ones who have what is considered
41:17 domain expertise right so they're the ones who deal with the representation of infrastructure that they're that they're
41:23 running all of their important business applications on i'm never going to understand it as well as they do um so
41:29 figuring out again how to work with them is something that is imperative to me and i encourage everybody in an
41:35 operational capacity who maybe has a data science team inside of your organization to find them
41:40 figure out if there's a way to ed's point that you can feed them some ops data right
41:45 it's it sounds like uh data science these data scientists are coming become
41:51 becoming almost like the most inner circle in in problem solving and then people start hovering around data
41:58 scientists to get this problem solved and this is similar to how we operate at nist for example i mean r d sits next to
42:06 product management but data science is right there cause data science needs both like you said the domain experts
42:13 and then some of the heavier r d muscle to really you know productize uh some of
42:18 those findings and things like that i wonder if marcel is still on the line uh
42:24 i'm still on the line yeah so that's that's uh
42:30 can you hear me yeah yes and now we can see you too i'm back away so
42:35 so i i would argue against that notion that data science is becoming the center
42:40 of gravity and because i think it's something similar happening um as we saw
42:46 with the evolution or the birth of the devops culture and sres when we had
42:53 developers and operational people like 20 years or 10 years ago and we had
42:58 those two camps when you threw your software over the wall to the ops people
43:03 and let them operate it nowadays we're looking for
43:08 devops people which are implemented by an sre which is
43:14 using tools of both worlds so the i think
43:20 the same should happen with the sre is now adopting ai and machine learning and
43:25 data science tooling and this this data science thinking to become a
43:31 data-driven sre person i think it's it's not just one group and one mindset of people um
43:38 orchestrating all the others and and talking to each other but it's it's
43:43 we we've we are we are we are embedded in data and we are uh the world is getting more and more complex so we need
43:50 to come up with tooling to understand and analyze that data and that's something that
43:56 each of us has to learn somehow and grow on on using these tools but otherwise
44:04 yeah we come back to the jobs question so that's that's actually a really good
44:11 uh point so so uh just so that the data science uh
44:16 isn't the privilege of a few and and to like kind of spread the message spread the tooling around the organization and
44:23 to like every developer and beyond uh how do how does one effectively uh
44:29 spread this kind of culture inside the organization uh like data
44:35 i mean the existence of data science in laggard organizations but also like like the benefits how how to utilize it
44:42 to to the you know best benefits there is actually a pretty good book about it
44:48 from the business perspective but but uh how do you see like what's the most effective uh way of building a culture
44:55 around utilization of machine learning and data science how do organizations get the most out of it
45:02 yeah i think there's a couple things to marcel's point earlier uh how to be able to consume it
45:09 it operators aren't developers and they're not data scientists and i don't think they want to be either one
45:15 right they want to be able to operate efficiently you need to have the tooling sets consumable for them in the right
45:20 way which means that they don't want to spend their lives learning how to do specific algorithms or having to prune
45:26 particular data sets they want to be able to have a set of tooling with the right set of interfaces for them to be
45:32 able to consume the data and get insights that's how you're going to get operators to adopt and be able to use
45:37 that i think today the space that that's being solved in is is a combination of open source and a combination of vendors
45:43 who are providing the right sets of of wrappers and consumption models because they understand their customers they
45:48 understand what they're looking for and they're trying to put glue those two sets of things together without necessarily coming up with proprietary
45:55 sets of of of tooling because i think that's the hard thing for many customers is is if
46:01 you go and talk with your data scientist teams they're probably adopting a set of frameworks from you know red hat or from
46:07 other entities that are trying to put together those open source tooling components and if you walk in as an
46:12 operator and say well i only know how to use x can you provide me the same interface that becomes a the big
46:18 challenge for the operator role versus what maybe your data scientist folks are doing internally so i think that's one
46:24 set of hurdles about how how folks can sort of you know understand where your operator
46:29 will understand what your boundaries are and yeah there's going to be some learn this is the job role change that we were talking about earlier you're going to
46:34 have to learn things that are outside of your core skill sets in order to be proficient but we had to do that all along anyway like anyone who was in
46:41 networking who didn't think you had to learn you know linux or learn windows or learn something else in order to be able to operate on the network
46:48 that's just a fallacy that's a lie you still had to do it you're still interfacing day to day on your mac or on
46:53 any other system to go admin those things so you have other additional skill sets this will be fit in that same
46:58 category where you're going to have to learn how to understand some base level sets of of you know
47:05 ai machine learning maybe some deep learning components that that fit in there at least to be able to have a good conversation with the teams around
47:11 what's going on and then also to be able to suss out what's important out of the data and information that you're being
47:17 provided out of the system right that's a behavior side am i actually getting something useful out of the system or
47:22 not and i think that's those those skill sets are going to be the ones that come onto your plate
47:28 because you're able to let go of the grind of the maybe the day-to-day operations change config management work
47:34 that hopefully the the automation is really sort of helping to to get off of your plate and not have to
47:40 deal with i don't know if that's a you know a good line of thought or not around that but that's sort of where my head is
47:47 at around it i think i think a on a on a company
47:52 level you have to adopt data as a product so it's not a
47:57 side effect that your company produces data and people have to use it to detect
48:03 bugs in your company but you are producing data and that means that you need
48:09 people and teams that have a product owner for the data that
48:16 you're producing and that can start with um things that add and add highlighted that you need to
48:24 go and talk to other people and understand their data and make it really easy for me as somebody not in that team
48:30 to access their data and not go through all the hoops there it must be effectively as easy as
48:37 launching up a browser and i can dive into the data there so as much as i can
48:43 launch google and end up on wikipedia and i have all the access to the internet we take that for granted nowadays but
48:50 even in red hat it's sometimes super super complicated to get the locks of some some certain system because uh they
48:56 are not treating data as a product so i think that's the first mindset on a company level that's uh you need to
49:03 do that transformation and that realization that data from each team is as important as an interface to that
49:11 team as and here's my change request but i also need to go to that team and say here's my here's my data request and
49:17 that must be fulfilled within minutes
49:23 yeah i built a little bit a little bit on both um from ed's perspective of like the
49:29 operator side of the world meet humans on the perceptual plane right
49:34 what i mean by that is improve the user experience overall and that bleeds a little bit into what marcel was highlighting as well which is like there
49:41 is something called a data user experience and what that can encompass depends on whatever data your system produces right
49:49 and you should have a data product manager that handles like that experience with that particular data set
49:55 but meeting humans on the perceptual plane is a socio-technical problem right it's not just social and it's not just
50:00 technical driving that mindset into your organization is going to take not only technology but
50:06 also culture shift right and figuring out what that means is going to be specific to the dna of the business
50:13 that you're working within um so that's not to say it's not impossible that's just to say that there are priors that
50:18 come with working inside of an organization and understanding those priors is important um and then lastly uh
50:26 again i'm a huge advocate of open source and open science data science and machine learning is empirically driven
50:31 what that means is i need to be able to prove to you that i did something that was useful um and then useful is based
50:37 on baselines or whatever it is that you're building that represents what means value to you what is your risk
50:42 function for your business right and then improving on those baselines um is important and being able to improve
50:49 and prove those results is like i said entirely empirically driven so open source and open science kind of just begets that
50:55 because you have to publish your your results in a way that other people are able to reproduce it right
51:00 so i think there's a lot of different angles that you could figure out how to take that with respect to building this practice inside of your organization but
51:06 it's important to make sure that it's not just a social and not just a technical problem
51:12 [Music] very good uh i know we're running a low on time but
51:18 before we go a couple more things one is a question from the audience a very interesting question just came from
51:25 walter does anyone think leadership in ai and ml uh like many things would come out of
51:32 darpa like it or not a lot of government standardization comes from u.s government defense
51:38 technologies what's what's the situation there anybody have any insight
51:46 anybody want to go i guess i can address part of it i i
51:53 think there's been a shift in in the um in how the evolution of the internet and
51:59 standards has has progressed over time and i think this is part of that evolution of of going from a centralized
52:05 you know darpa influencing you know open standards bodies the itf as an open collaboration identity um
52:12 and others and it's moving away towards much more of the democratization of it
52:18 through open source of innovation through open source and not necessarily through standardized formal bodies and i
52:23 think um i think this is an example where you may not necessarily see
52:29 an organization like darpa try to come out with uh sort of the standard sets around leadership around
52:36 ml necessarily in the technology space that may not be true from a governmental
52:41 perspective around compliance conformance regulatory stuff
52:47 that happens i think there's going to be a whole space and you know microsoft has made some very public statements about what marcel
52:53 was talking about earlier about you know face recognition facial recognition how that should be used what are the social
52:59 implications of that for data privacy and a bunch of other things and that's the whole different discussion that's
53:04 not even around i think aiopside but i think you aren't necessarily going to see the standards come out that way i
53:10 think the standards are going to happen based off of what at henry's point was earlier how open are the data sets how
53:16 how much is community willing to contribute to move uh the industry forward to have the right sets of data
53:21 models that are that can be produced and the right data sets that can be produced and shared in the right way to allow
53:27 everyone to sort of validate what's going on and to agree about what those standards need to be and i don't necessarily think that
53:33 you know i mean the defense industry can definitely contribute but i don't think they're going to be the only contributor
53:39 nor set the standard for what's happening in that area i could be completely wrong so it's my personal
53:44 opinion i put that out and i'm going to punch it to ed henry to just sort of reply back to that
53:49 [Laughter] darpa is an interesting beast they dabble in a lot of technologies
53:56 that aren't necessarily just defense related either um in network standardization yes some
54:01 things have come out of darpa with respect to maybe some of the security standards and interworkings with nist
54:07 and things along those lines but i would actually make the argument that the ietf has largely driven network
54:12 standardization for a very long time and that consists of both industry representation along with research
54:19 representation and government representation so i do think that some advances will come
54:24 out of that leadership is a relative term it's pretty clear right now that leadership exists in industry at least in the perceptual
54:31 space of machine learning computer vision natural language processing things along those lines but make no mistake there's probably darpa funds
54:36 behind some of those labs as well i don't have a direct answer to that i think just because of the sheer breadth
54:43 and depth at which darpa likes to explore enterprises and by enterprises i
54:49 mean people who want to submit grant applications that can then go and do some sort of research that darpa might
54:54 find valuable all right and i might find a question
55:01 yeah go exploration you know so instead of
55:06 mentioning darpa i think the linux foundation just launched two years ago an ai and data initiative
55:14 and they came out with the first gpl equivalent and licenses for data so
55:19 i think there's there's a lot of groundwork still to be done and the most traffic and velocity i'm seeing
55:27 there is in that domain so go to lfaidata.foundation
55:32 and you see something going on there and
55:38 we only have a few minutes left but but uh in a nutshell uh just for the aspiring uh
55:46 you know semi-data scientist in us like network engineers and networking managers uh
55:52 what would be your goal to place like if there was one resource of information where one one should go to uh to get
55:59 excited and learn more um about aiops what what would be your choice besides
56:05 mis.com of course and try out our stuff and we'll see where it's productized today uh but but if you want to think a
56:12 tinker uh more with the data science tools and things like that
56:20 not necessarily even tools but also books uh websites further reading any any kind of
56:26 further uh you know resources people could take from my perspective
56:33 any type of social media i would encourage the scientific community has taken uh to
56:39 twitter quite a bit uh if you're interested in the theoretical side of things but also the operational side of things having been on something like
56:46 twitter for almost a decade if not longer at this point there's a huge representation of
56:51 individuals that are working on a broad number of things generally and again this idea of open source open
56:58 science i haven't met a community yet um in the world of machine learning and data science that isn't trying to
57:03 embrace people who are just interested in the technology all the way through to doing fundamental research so i just
57:09 encourage anybody to pick your favorite social media platform find some work that you're interested in doing some
57:14 problems that you're interested in helping solve and i think just through that natural kind of exploration process you'll find a set of individuals that'll
57:21 help you kind of grow yourself very good any uh if you had to name a couple of uh twitter accounts to follow
57:27 what would be your favorites of course uh in addition to ed henry um well i tend to lean on kind of the
57:35 theoretical side of things um but i really that's on the spot man i think
57:41 i follow like 900 people on twitter right now um there are you know depending on what it is that you
57:47 uh are interested in you know what i could recommend find my twitter handle and just look at people that are following me or following or i'm
57:54 following or part of lists or things along those lines i don't have an answer for that off the top of my head
58:00 that's really really good uh i usually tell people the same thing if you want to know wi-fi geekery just go find me
58:06 and you know the people i follow what about marcel what do you think
58:12 if you have to point people to one resource so so i can i can drop a shameless flux
58:18 here um on our operate first initiative where we try to democratize and
58:23 come up with an open source equivalent to operations and building a completely transparent cloud setup
58:32 from workloads into the data center back end that's on operate
58:37 dash first dot cloud that's the url but in terms of
58:44 so i like reading books and the last book that i really that really struck me and was really inspirational was by jeff
58:51 hawkins like steve hawkins but jeff hawkins he's a neuroscientist and he wrote a book called on intelligence and
58:59 he has a really drawn painted a really nice picture of the brain which is just
59:05 consuming metrics in the end and
59:10 so that gave me hope that there will be some general artificial intelligence at some
59:16 point if our if if our brain can do it we should be able to repeat it with
59:21 machines that's that's a very well written book on intelligence
59:27 god it goes on my list for sure and mr horley
59:34 yeah probably the easiest thing is just if you're interested on the off side you're interested about the impact of ai
59:39 on ops just follow the hashtag for you know the hashtag aiops on twitter and you're gonna see a whole
59:45 stream of everyone who thinks they're applicable and then just go start pruning through for what you think is the best way so i agree with that henry
59:51 but most of the stuff that i keep track of i keep track of on twitter because it's the easiest way to consume it fast
59:56 i i do i do think red hat has some good information available up on their site too to sort of peruse
1:00:01 through and sort of get started uh if you don't understand anything about the operation side about what ansible can do
1:00:08 about what some of their product sets can do in terms of data ingestion and getting data insights if you never run
1:00:13 prometheus before go you know those sorts of things you need to you need some place to get started to go get
1:00:19 the software installed and play around with it so those are good guidelines to be able to go find that stuff and that's sort of and build a lab go tinker i mean
1:00:26 most of the stuff is available as as cloud resources and most of the major cloud platforms you can go get it built pretty quickly and and play around with
1:00:33 sample data sets that might be the other way to to sort of jump on and get started if that's something of interest
1:00:38 for yourself absolutely and and we have uh quite a bit of course
1:00:46 material on ai ups a ai and networking uh if you go to news.com webinars we for
1:00:52 example had a three-part ai ups uh webinar that we did with walmart and
1:00:57 some of the mark from walmart and some of the other guests uh you know concerning different matters of ai ups
1:01:04 um and uh in general nist.com webinars and on our website in general there's
1:01:10 there's a lot of stuff there but um and if you're if you're into networking wi-fi geekery uh follow my twitter
1:01:16 handle as well it's it's my first name last name altogether and uh if you guys don't mind if you can just put your
1:01:22 twitter handles also or or your linkedin pages what have you on on the chat
1:01:28 there i don't know if uh actually that's visible to everybody but
1:01:34 it's probably not anyway um you'll you'll find us on the on the signing page for example you can
1:01:41 find our our twitter handles and on twitter and find us uh any final words anybody before we sign out i know
1:01:48 we're a couple of minutes overdue already uh who wants to raise raise your hand and
1:01:53 give a final statement on where where are we today with ai ops and and where do we go from here
1:02:05 i'll i'll close that by saying it's it's it's not something that you guys should be scared of it's something that you guys should embrace uh uh this will make
1:02:11 your life easier over the long haul your job will actually get more interesting you get to work on more interesting problems and not necessarily have the
1:02:17 day-to-day grind stuff that goes on but it'll be a journey it's not overnight and uh you know
1:02:23 you know start doing your learning now so it's not it's it's not scary that's that's
1:02:29 what i would give us advice so i'm just watching the series um
1:02:35 season 3 of westworld so i can only give one advice be friendly to our ai
1:02:40 overlords
1:02:45 that already became clear in the first last episode of season one right
1:02:51 but with that i i guess it's time to to end the show thank you so much everybody
1:02:56 for joining thank you panelist marcel ed and ed uh really appreciate you guys coming over
1:03:04 taking your time and sharing your expertise i hope this was useful to uh
1:03:09 to the audience and uh i hope to see you again soon
1:03:14 thank you thank you take care everyone bye
1:03:22 cheers
1:04:05 you